Data Preprocessing Techniques: 6 Steps to Clean Data in Machine Learning
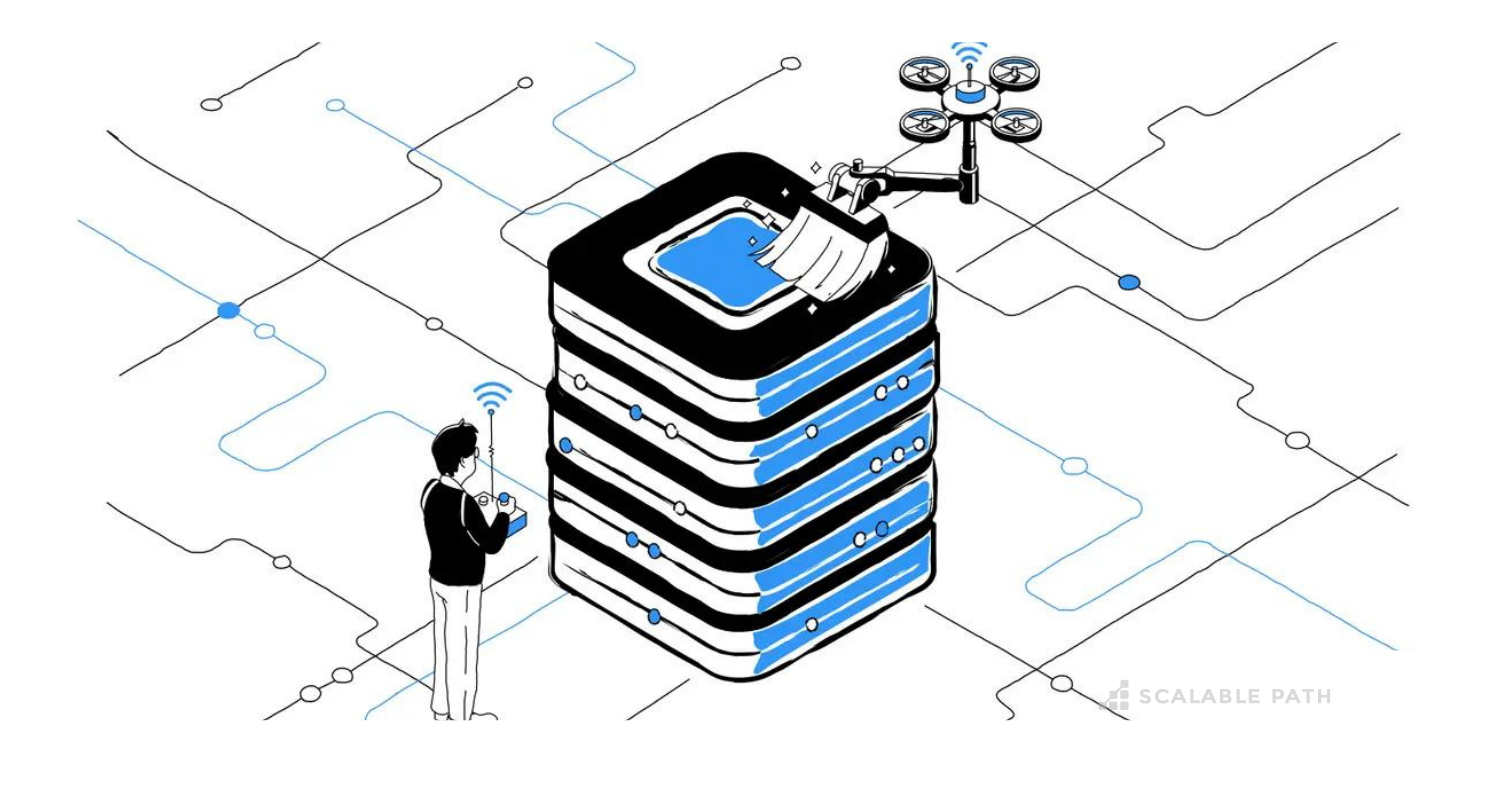
The data preprocessing phase is the most challenging and time-consuming part of data science, but it’s also one of the most important parts. If you fail to clean and prepare the data, it could compromise the model.
“If 80 percent of our work is data preparation, then ensuring data quality is the important work of a machine learning team.”
– Andrew Ng
When dealing with real-world data, Data Scientists will always need to apply some preprocessing techniques in order to make the data more usable. These techniques will facilitate its use in machine learning (ML) algorithms, reduce the complexity to prevent overfitting, and result in a better model.
With that said, let’s get into an overview of what data preprocessing is, why it’s important, and learn the main techniques to use in this critical phase of data science. Here’s everything we’ll cover in this guide:
Table Of Contents
What is Data Preprocessing?
After understanding the nuances of your dataset and the main issues in the data through the Exploratory Data Analysis, data preprocessing comes into play by preparing your dataset for use in the model.
In an ideal world, your dataset would be perfect and without any problems. Unfortunately, real-world data will always present some issues that you’ll need to address. Consider, for instance, the data you have in your company. Can you think of any inconsistencies such as typos, missing data, different scales, etc.? These examples often happen in the real world and need to be adjusted in order to make the data more useful and understandable.