Agentic AI: Building Intelligent Workflows [Guide]
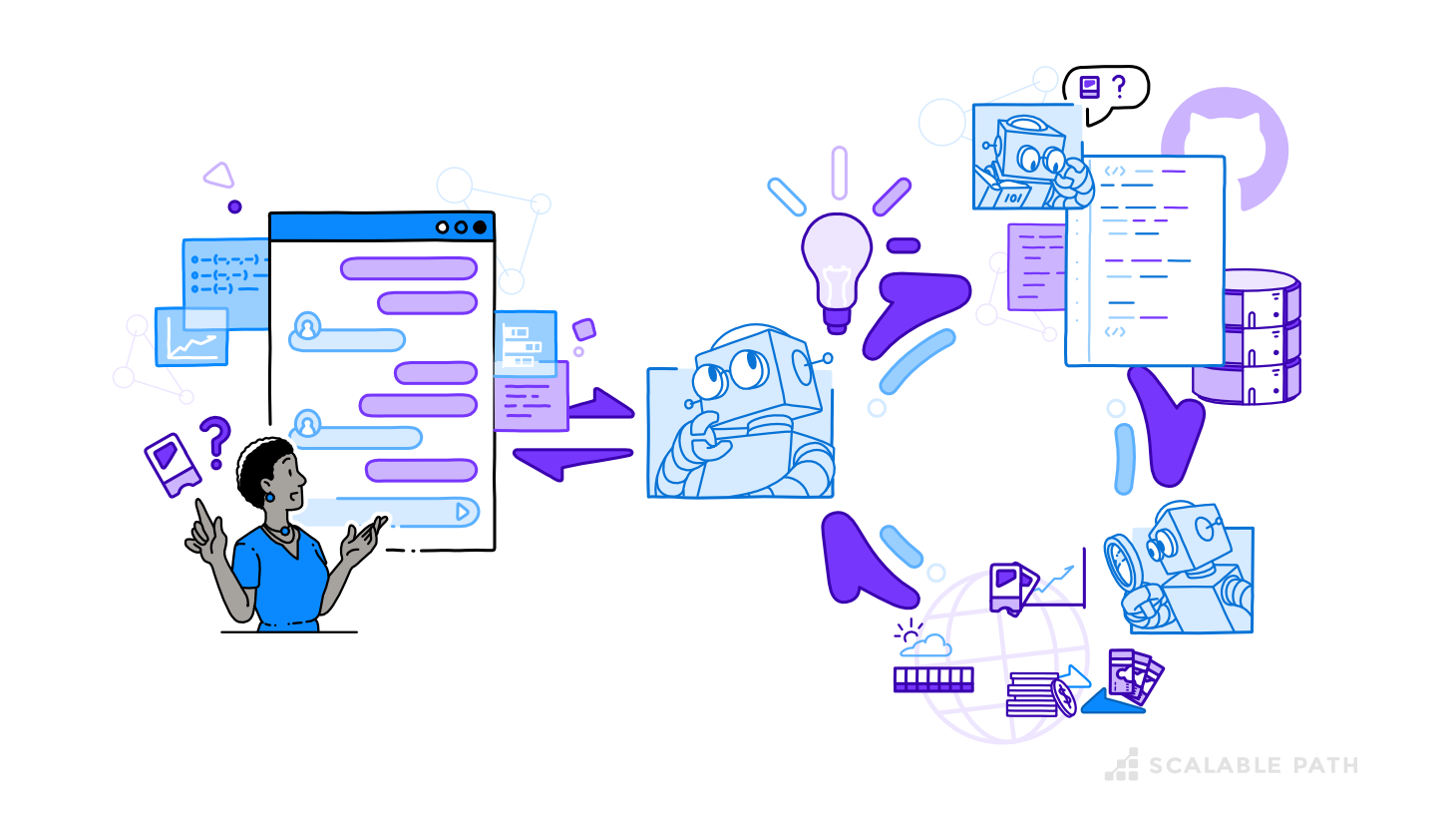
AI agents are everywhere in conversations lately, and for good reason. Today, I’m excited to dive into this groundbreaking innovation: agentic workflows.
My personal interest in agentic workflows has grown in recent months, due in large part to a few articles I’ve written recently. One was a piece evaluating the real-world efficacy of AI agents (ChatDev, SWE-Agent, and Devin). The second was a deep dive into Devin AI, the tool I found most compelling from my initial research.
So, why are AI agents so fascinating to me? Unlike tools like ChatGPT, which follow a straightforward prompt-and-reply interaction model, AI agents are designed for more dynamic and context-aware engagement. Not only do they understand the broader context of a task, but can also plan strategies, critique their own outputs, and adapt their responses to user inputs in real time.
While this method of interaction is compelling on its own, I wanted to push the boundaries further and explore how agentic workflows can be used to create specialized AI tools. Tools capable of integrating with systems across your tech stack, perform complex, multi-step tasks, and deliver results that are ultimately more powerful than what traditional AI can achieve.
So, what exactly sets AI agentic workflows apart, and how do we go about building one? In this article, I’ll break down the key advancements that made agentic workflows possible, explore their real-world potential, and provide practical insights into how you can create and leverage these systems.
Table Of Contents
- Key AI Advancements That Led to Agentic AI
- Three Key Characteristics of AI Agentic Workflows
- The Problem With Traditional AI Tools: Zero-Shot Prompting
- Building an AI Agent: Key Design Patterns
- Retrieval-Augmented Generation (RAG) and AI Agentic Workflows
- Building an Agentic Workflow: Tech Stack and Key Components
- Challenges and Considerations of AI Agentic Workflows
Key AI Advancements That Led to Agentic AI
To me, the current AI revolution feels a lot like the JavaScript framework gold rush of the mid-2010s. It felt like new tools and concepts were popping up daily, each claiming to redefine the game. What we didn’t always see then, however, was the years of innovation and behind-the-scenes experimentation that made these new JS frameworks possible.
The same thing is true today. AI agents haven’t evolved out of nowhere – there were some key innovations that enabled us to get where we are.